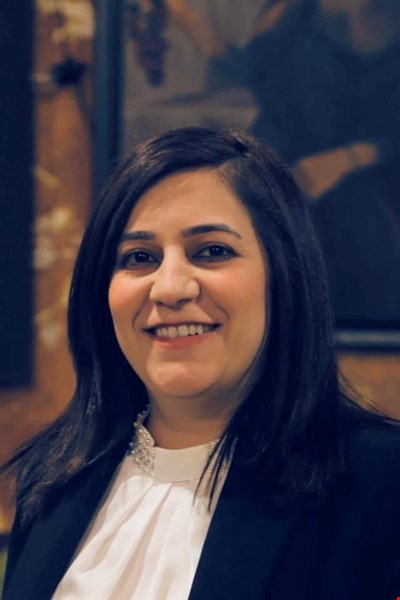
Miss Roba Zayed
PhD Student
Research area(s)
Big Data AI System for Air Quality Prediction
My research focuses on the critical field of air quality prediction and management, employing state-of-the-art technologies like big data analytics, artificial intelligence, and hybrid intelligent modeling. The primary objective of the research is to develop advanced predictive models that accurately measure air quality levels in urban environments.
Cities are facing increasingly complex air quality challenges due to various air gases and components. To address these issues, my research incorporates innovative methodologies, leveraging the power of big data and AI. The predictive models produced by this research offer precise and data-driven insights, empowering decision-makers across multiple sectors to make informed choices.
Beyond environmental implications, my findings contribute to sustainable development and economic growth while emphasizing the preservation of social values within urban areas. The multi-input multi-output hybrid model deployed in this research achieves high accuracy for hourly time-series data, ensuring the credibility and reliability of the predictive models.
By striving to close the gap in high big-data prediction accuracy, this research aims to contribute to the establishment of a more standardized air-quality index. Ultimately, the goal is to combat air pollution and create healthier and more sustainable living environments.
Overview of Research from Two Publications
- Publication: "Big Data AI System for Air Quality Prediction" (ICONDATA Conference)
Abstract: The first publication focuses on the development of a machine learning model to predict air quality levels in cities. The researchers address the increasing complexity of air quality prediction due to various air gases and components. The paper aims to provide accurate air quality predictions to support data-driven decision making in different sectors related to sustainable development, economic growth, and social values. The research contributes to air quality policy formulation with the goal of mitigating global consequences of pollution and promoting cities' sustainable development. The study utilizes big data and artificial intelligence techniques to predict air quality, and the resulting model showcases promising accuracy in predicting air quality levels.
Publication Details:
- Conference: ICONDATA
- Location: Turkey (presented online)
- Date: June 4th - 6th, 2021
- Authors: Roba Zayed, Maysam Abbod
- Abstract Published in: ICONDATA proceeding (Abstract_Book_ICONDATA2021.pdf)
- Selected as one of top 20 paper in the conference and published in "Data Science and Applications" journal.
Reference: Zayed, R. and Abbod, M. (2022) “Big Data AI System for Air Quality Prediction”, Data Science and Applications, 4(2), pp. 5-10. Available at: http://www.jdatasci.com/index.php/jdatasci/article/view/63
2. Publication: "Hybrid Intelligent Modelling for Air Quality Prediction Deep Learning and Markov Chain Unconventional Framework" (UKSim-AMSS 24th International Conference)
Abstract: The second publication introduces an innovative prediction model for measuring air quality in selected cities. The researchers propose a multivariate hybrid Markov-switching dynamic model using a multi-state transition method and a Deep Neural Network within a niche experimental framework. The study is part of applied big-data AI research with the aim of providing a reliable air-quality index using the hybrid model. The research contributes to filling the gap in high big-data prediction accuracy for hourly frequency data and aims to establish a more standardized air-quality index by comparing results in two selected cities: London and Jordan.
Publication Details:
- Conference: UKSim-AMSS 24th International Conference
- Location: Cambridge, United Kingdom
- Date: April 13th - 15th, 2022
- Authors: Roba Zayed, Maysam Abbod
- Published in: International Journal of Simulation - Systems, Science & Technology, 2022, Vol. 23 Issue 1, p1-6.
Reference: Zayed, R. and Abbod, M. (2022) “Hybrid Intelligent Modelling for Air Quality Prediction Deep Learning and Markov Chain Unconventional Framework, UKSim, Cambridge. Available at: Microsoft Word - paper3.docx (ijssst.info)
Both of these publications showcase the researchers' dedication to advancing the field of air quality prediction and their commitment to utilizing cutting-edge technologies and methodologies to address the challenges associated with air pollution and its impact on society and the environment.
#AirQualityResearch #DataScience #AIinEnvironment #EnvironmentalScience #PredictiveModeling #SustainableDevelopment #CleanAir #UrbanEnvironment #ClimateAction #ResearchInnovation #BigData #ArtificialIntelligence #DataAnalytics #EnvironmentalImpact #AirPollution #ResearchWork #GreenTech #DataDrivenDecisions #SustainabilityResearch #AirQualityIndex #ScienceAndTechnology
Research Interests
Big Data, AI, Machine Learning, Air Quality, Prediction