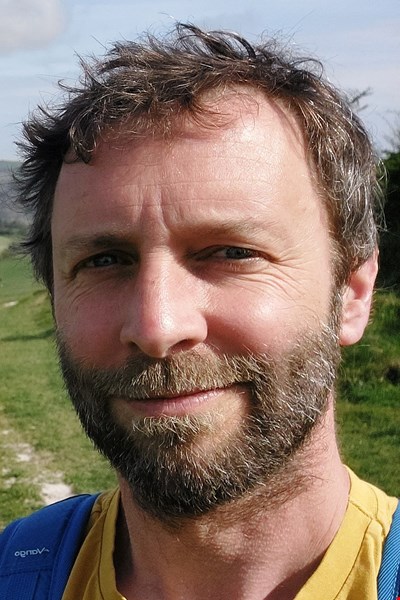
Dr Allan Tucker
Reader
Wilfred Brown Building 218
- Email: allan.tucker@brunel.ac.uk
- Tel: +44 (0)1895 266933
Summary
Allan Tucker is Reader in the Department of Computer Science where he heads the Intelligent Data Analysis Group consisting of 17 academic staff, 15 PhD students and 4 post-docs. He has been researching Artificial Intelligence and Data Analytics for 21 years and has published 120 peer-reviewed journal and conference papers on data modelling and analysis. His research work includes long-term projects with Moorfields Eye Hospital where he has been developing pseudo-time models of eye disease (EPSRC - £320k) and with DEFRA on modelling fish population dynamics using state space and Bayesian techniques (NERC - £80k). Currently, he has projects with Google, the University of Pavia Italy, the Royal Free Hospital, UCL, Zoological Society of London and the Royal Botanical Gardens at Kew. He was academic lead on an Innovate UK, Regulators’ Pioneer Fund (£740k) with the Medical and Health Regulatory Authority on benchmarking AI apps for the NHS, and another on detecting significant changes in Adaptive AI Models of Healthcare (£195k). He is currently academic lead on two Pioneer Funds on Explainability of AI (£168k) and In-Silico Trials (£750k). He serves regularly on the PC of the top AI conferences (including IJCAI, AAAI, and ECML) and is on the editorial board for the Journal of Biomedical Informatics. He hosted a special track on "Explainable AI" at the IEEE conference on Computer Based Medical Systems in 2019 and was general chair for AI in Medicine 2021. He has been widely consulted on the ethical and practical implications of AI in health and medical research by the NHS, and the use of machine learning for modelling fisheries data by numerous government thinktanks and academia.
Responsibility
Selected Invited talks • (KEYNOTE) How to Deal with Privacy, Bias & Drift in Synthetic Primary Care Data, Alan Turing Institute 2023 • (KEYNOTE) Safe AI in Healthcare, AI meets Head and Neck Oncology 2022 • Transparency and the Importance of Explanation, International Congress on the Exploration of the Sea ASC 2019 • How to Analyse Big Data, The Royal Society of Medicine (KEYNOTE), 2014 • Artificial Intelligence, Useful Tools or Robot Overlords, Skeptics in the Pub, London 2014 • Machine Learning Approaches to Modelling Fisheries Data, Plymouth Marine Laboratory, 2013 • The Intelligent Data Analysis of Natural Process Data, Harbin Institute of Technology, China (also to Makerere University School of Computing and IT, Uganda via Skype) 2012 • Integrating marine biomass data using foodweb expertise, British Ecological Society, 2012 • Probabilistic Models for Understanding Ecological Data: Case studies in Seeds, Fish and Coral, Computational Sustainability, 2012 (KEYNOTE) • Bioinformatics tools in predictive ecology: applications to fisheries, The Royal Society 2011(KEYNOTE)
Program Committees I am / have been on the program committee for many international conferences including: • Knowledge Discovery in Databases - KDD (Research), • AI in Medicine (Board Member), • American Association for AI conference - AAAI, • International Joint Conference in AI - IJCAI • General chair for the symposia of IDA 2013 (Council Member) and for AI in Medicine 2021 (Board Member),
I review for numerous journals including: • Nature Protocols, • PLOS ONE, • IEEE Transactions on Evolutionary Computation, • AI in Medicine, • Journal of Biomedical Informatics (on Editorial Board)
Newest selected publications
Draghi, B., Wang, Z., Myles, P. and Tucker, A. (2024) 'Identifying and handling data bias within primary healthcare data using synthetic data generators'. Heliyon, 10 (2). pp. 1 - 15. ISSN: 2405-8440 Open Access Link
Rotalinti, Y., Myles, P. and Tucker, A. (2024) 'Predicting Performance Drift in AI Models of Healthcare Without Ground Truth Labels'. Springer Nature Switzerland. pp. 167 - 178. ISSN: 0302-9743
Nezhad, FH., Rotalinti, Y., Myles, P. and Tucker, A. (2023) 'Privacy Assessment of Synthetic Patient Data'.2023 IEEE 36th International Symposium on Computer-Based Medical Systems (CBMS). L'Aquila, Italy. 22 - 25 June. IEEE. pp. 1 - 6. ISSN: 2372-918X
Khoshravan Azar, A., Draghi, B., Rotalinti, Y., Myles, P. and Tucker, A. (2023) 'The Impact of Bias on Drift Detection in AI Health Software'.AIME 2023 : 21st International Conference of Artificial Intelligence in Medicine. Portorož, Slovenia. 5 - 15 June. Springer Nature Switzerland. pp. 313 - 322. ISSN: 0302-9743
Myles, P., Ordish, J. and Tucker, A. (2023) 'The potential synergies between synthetic data and in silico trials in relation to generating representative virtual population cohorts'. Progress in Biomedical Engineering, 5 (1). pp. 1 - 5.